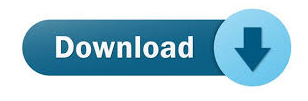
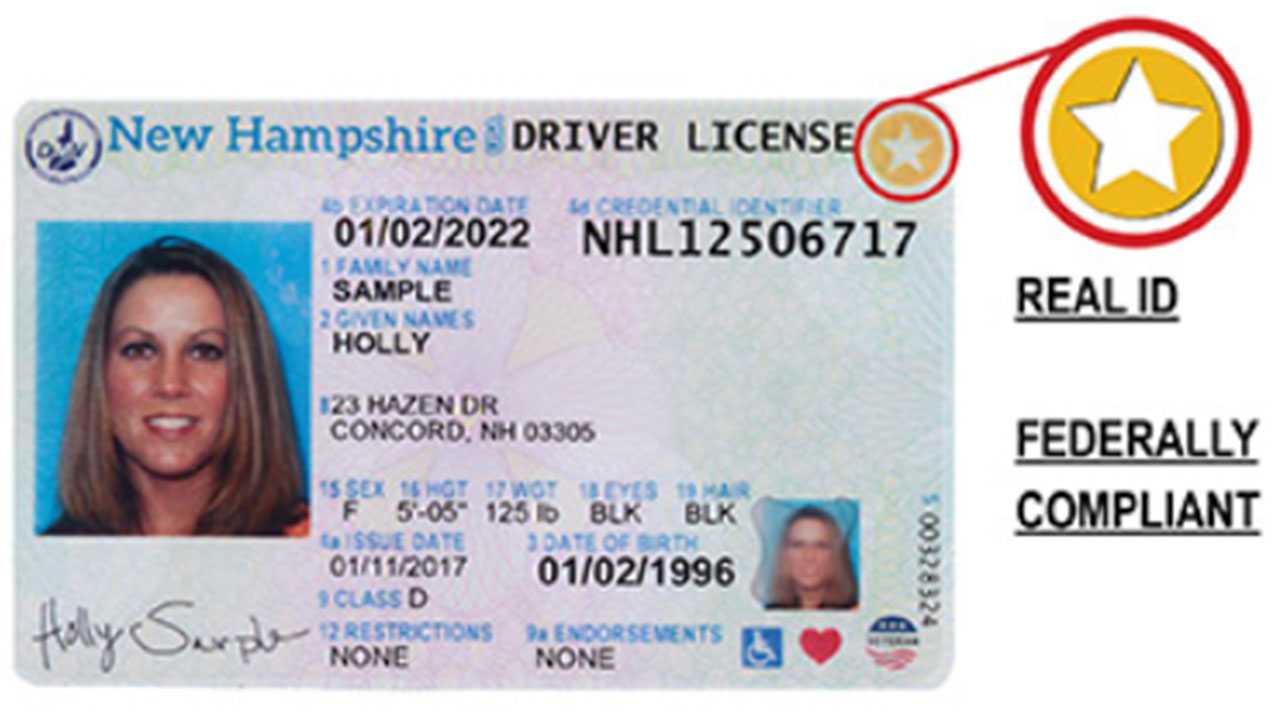

Experimental results on the PASCAL VOC, COCO, and ILSVRC datasets confirm that SSD has competitive accuracy to methods that utilize an additional object proposal step and is much faster, while providing a unified framework for both training and inference. This makes SSD easy to train and straightforward to integrate into systems that require a detection component.

SSD is simple relative to methods that require object proposals because it completely eliminates proposal generation and subsequent pixel or feature resampling stages and encapsulates all computation in a single network. Additionally, the network combines predictions from multiple feature maps with different resolutions to naturally handle objects of various sizes. At prediction time, the network generates scores for the presence of each object category in each default box and produces adjustments to the box to better match the object shape. Our approach, named SSD, discretizes the output space of bounding boxes into a set of default boxes over different aspect ratios and scales per feature map location. We present a method for detecting objects in images using a single deep neural network. All the extracted information will help historical and societal studies over the photo archive. We further analyze the similarities and differences between the photographers using the features extracted from the photographer classifier network. Furthermore, we managed to train a neural network that can successfully recognize the photographer from some of the photos, which shows that such photos are indeed characteristic for certain photographers. We were able to find some special characteristics for different photographers in terms of their typical photo content and photo types (e.g., close-ups vs. Specifically, we analyze prominent Finnish World War II photographers, who have captured high numbers of photographs in the publicly available SA photo archive, which contains 160,000 photographs from Finnish Winter, Continuation, and Lapland Wars captures in 1939-1945. In this paper, we demonstrate the benefits of using state-of-the-art machine learning methods in the analysis of historical photo archives.
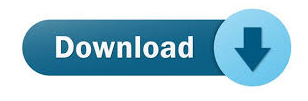